
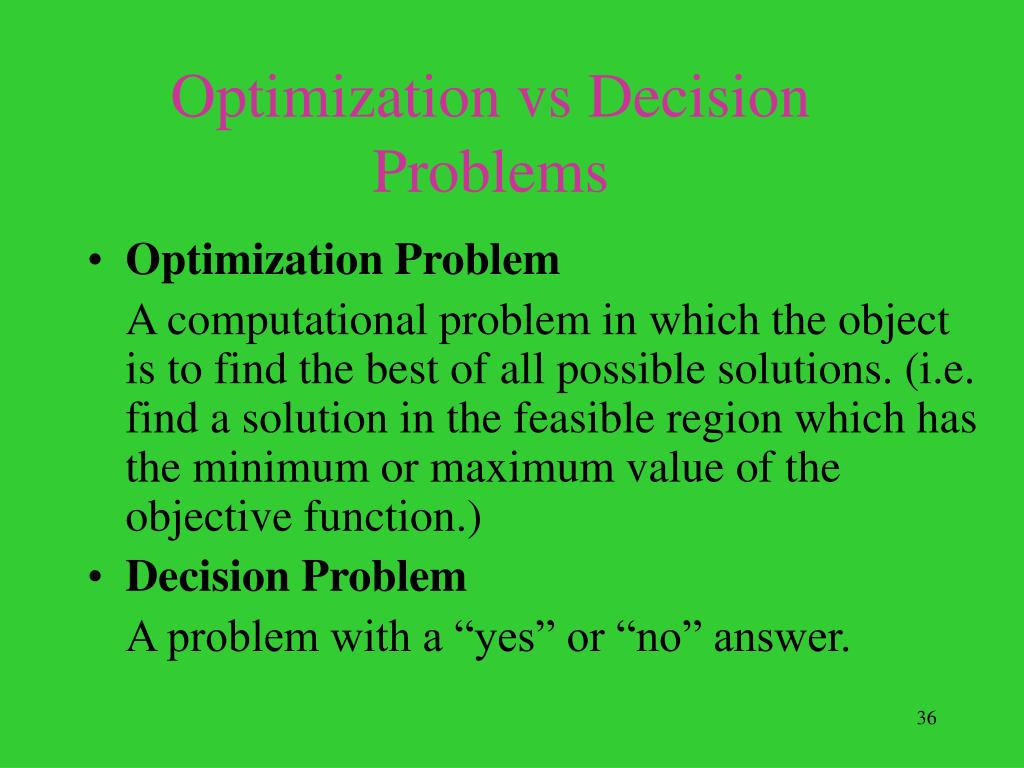
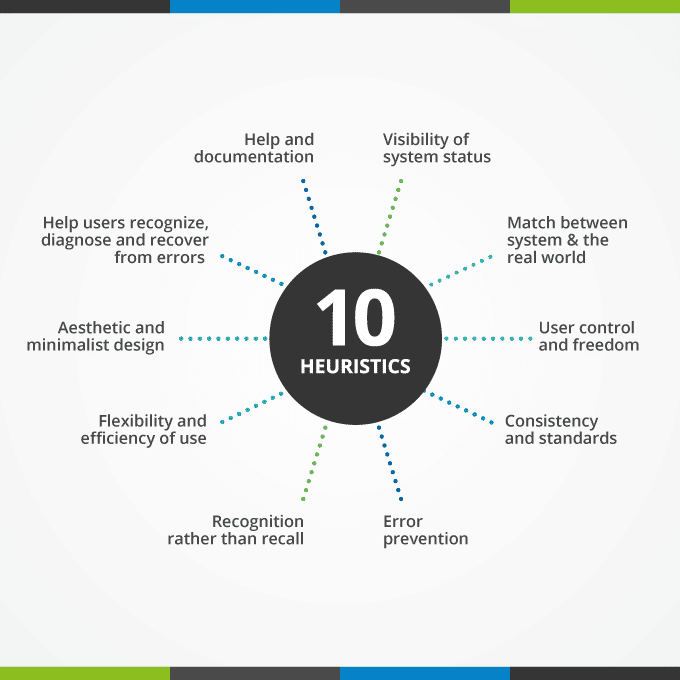
However, the solution found is not the optimal solution. Notice that the algorithm did not expand a single node that is not on the path to the solution. We continue to do so until we reach the goal. Looking at our heuristic values table, we determine that C is the closest to the goal, so we expand it and add its successors to the frontier. We first expand our initial node A, and we get three successor nodes B, C and D. Still, I find it easier to superimpose the search tree onto the state graph to demonstrate better how the frontier is expanded closer and closer towards the goal. The algorithm will create a search tree internally, just like uninformed searches. So a greedy best-first search is a best-first search where f(n) = h(n).Īs an example, we will look for a path from A to Z using the greedy approach. And recall that a best-first search algorithm will pick the node with the lowest evaluation function. Greedy Best-first SearchĪ greedy best-first search is a form of best-first search that expands the node with the lowest heuristic value or, in other words, the node that appears to be the most promising. It is optimistic because a straight line between two points will always be less than or equal to any other path. And we use that distance as an estimate to evaluate a node. Our heuristic will return the straight line distance between any node and the goal from that map. Recall our fictional map of towns from the last article, for which we will create an example of an optimistic heuristic function. If the heuristic is too high, then we don’t explore nodes, and we might miss a low-cost solution, but if the estimate is too low, then we tend to expand nodes and then later discard them when the actual cost for the node starts adding up. We typically want heuristics that underestimate the actual cost ( optimistic heuristic ) rather than overestimating ( pessimistic heuristic ). It uses domain-specific clues to estimate the cheapest path from the given node to a goal. Heapq.heappush(frontier, (f(child), next(counter), child))Ī heuristic function will take a node and evaluate how close it is to the solution. Heapq.heappush(frontier, (f(root), next(counter), root))Īnd f(child) >= explored.path_cost: :param f: Callable evaluation function that takes in 1 argument NodeĬounter = unt() # tie breaker for heapq :param problem: Implemented Problem class To avoid duplicates in the frontier, use an A* search with a The frontier if that node has not been reached before, or if the new path hasĪ lower cost. This algorithm will track reached/explored nodes and will insert a node into In the frontier with the lowest f(n) value. Uses a priority queue to always explore nodes Takes in an implemented `Problem` and returns a `Node` once a solution isįound or `None` otherwise. def best_first_search(problem: Problem, f: Callable) -> Optional: By employing different evaluation functions, we get different specific algorithms, which we discuss in this article. Each child node is added to the frontier if it has not been reached before or is re-added if reached from a less costly path. On each iteration, we choose a node on the frontier with a minimum f(n) value, return it if its state is a goal state, and otherwise expand it to generate child nodes. Best-first SearchĪ very general approach in which we choose a node, n, with minimum values of some evaluation function, f(n). The idea is that while breadth-first search spreads out in waves of uniform depths (depth 1 -> depth 2 -> … ), best-first search spreads out in waves of uniform evaluations ( f(n) ). This article will see how we can pick more promising paths first and put aside the least likely ones for last, known as a best-first search or uniform-cost search. In the last article, we saw how a problem-solving agent could use search trees to look ahead and find a sequence of actions that will solve a problem, but we faced a drastic issue: uninformed search trees grow too fast!
